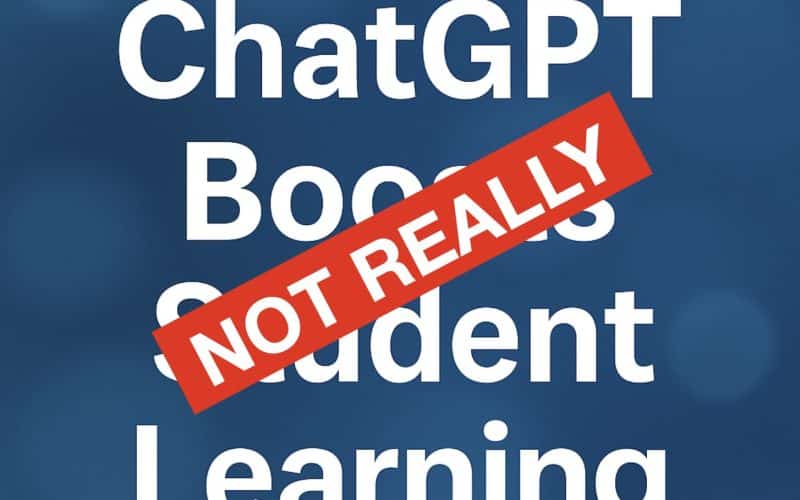
Remember the drama around the Microsoft and Carnegie Mellon Study which made headlines saying AI was making us dumb? A new study by researchers Jin Wang and Wenxiang Fan – who analyzed 51 studies on ChatGPT in education – seems to contradict the fearmonger headlines. You will further down the article understand why we put seems in italic.
Their meta-analysis – published in May 2025 – should mark a major shift in how schools should approach AI. Rather than asking if AI should be used in classrooms, the question now is how to use it effectively.
Their study, published in Nature’s Humanities and Social Sciences Communications section, directly addresses the rising interest in AI tools. It also addresses the concerns about screen time, equity, and learning quality.
However, not everybody agrees with what is published in this study. Ilkka Tuomi, Chief Scientist at Meaning Processing Ltd., for instance has serious doubts. But let’s first watch the video below to see what the study is about, followed by critical remarks on the methodology used.
- 1 AI and Learning Outcomes – But Are the Meta-Analysis Claims Reliable?
- 2 The Use of Hedges’ g in The Meta-Analysis by Jin Wang and Wenxiang Fan is Off (In this Study)
- 3 Major Concerns with the Meta-Analytic Framework
- 4 Misinterpretation Risks: What Does g = 0.867 Actually Mean?
- 5 Misleading Generalizations by Context
- 6 Support for Higher-Order Thinking?
- 7 Why These Effect Sizes Lack Reliability
- 8 Summary Table: Results Without Context Don’t Equal Truth
- 9 Complete Lack of Methodological Control,
AI and Learning Outcomes – But Are the Meta-Analysis Claims Reliable?
The recent meta-analysis by Jin Wang and Wenxiang Fan—analyzing 51 studies on ChatGPT in education – was widely shared as evidence that AI improves learning outcomes. The findings, published in Nature: Humanities and Social Sciences Communications, gained traction among educators and policymakers eager to explore AI integration in classrooms.
However, as the study circulated, critical voices began to emerge. Among the most vocal is Ilkka Tuomi, Chief Scientist at Meaning Processing Ltd., who questions the methodological integrity of the meta-analysis. His concerns point to deeper issues with how evidence is selected, interpreted, and presented in fast-moving AI-in-education research.
Here’s his initial comment on LinkedIn, where he isn’t exactly holding back: “That’s why I’ve called these studies junk.” Adding: “Not a very polite thing to do, but maybe a decent antidote is needed in the circulation.”
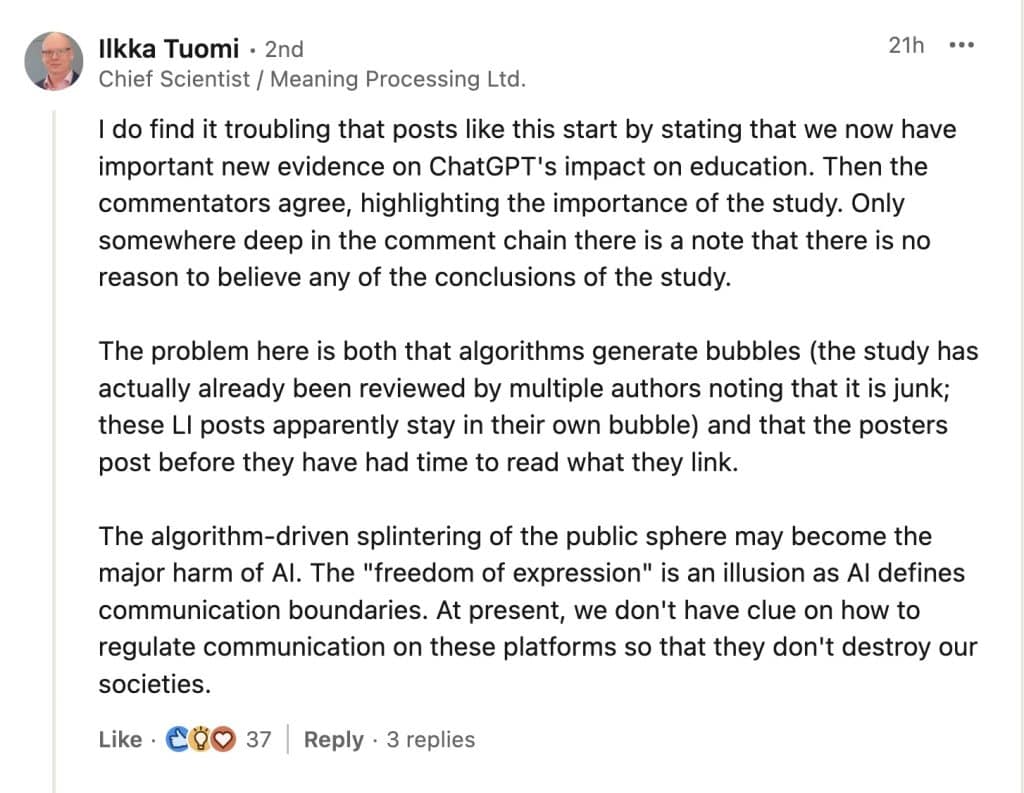
It was followed up a day later by this more elaborated LinkedIn update.
This post was again followed up by this update on LinkedIn where he adds extra context. Tuomi points to a fundamental flaw: the assumption that randomized or quasi-experimental studies – when grouped across wildly different settings – can reveal a causal impact. “No amount of computation can generate valid results if the populations differ and the outcomes are ill-defined,” he explains.
According to Tuomi, the study’s review process reduces thousands of articles to just 51, using keyword filters like ‘ChatGPT’ and ‘educ*’ – without clear controls on study quality or population consistency. “Half of the studies have group sizes under 35, some claim to make statistically valid conclusions based on groups with 12 students.”
“RCTs (Randomized Controlled Trials) only prove effects in specific contexts. Generalizations are not valid.” His critique isn’t just about one study – it’s about how we evaluate evidence in an era of fast science and faster headlines. Note that the RCTs are strong on internal validity but weak on external generalizability. In short: the generalization of results is the issue – not the RCT method itself.
The result is what Tuomi calls a “garbage in, gold out” effect, masked by statistical polish and a rush to publish.
The Use of Hedges’ g in The Meta-Analysis by Jin Wang and Wenxiang Fan is Off (In this Study)
Wang & Fan (2025) use Hedges’ g – a common statistical metric in education research – to calculate effect sizes across 51 studies evaluating ChatGPT’s impact on student outcomes. Hedges’ g is intended to standardize results by accounting for mean differences and pooled standard deviations, with a correction for small sample bias.
In theory, this metric allows researchers to:
- Standardize diverse study outcomes
- Adjust for small group sizes
- Provide cross-study comparability
Yet in the case of this particular meta-analysis, the application of Hedges’ g does not resolve core validity issues – instead, it obscures them.
To avoid any misunderstandings, the mathematical use of Hedges’ g is technically correct. The interpretive problem lies in applying it across weak or heterogeneous studies without quality filtering.
Major Concerns with the Meta-Analytic Framework
Wang & Fan do not report whether included studies were peer-reviewed, randomized, or sufficiently powered. The study fails to apply even basic quality screening or scoring methods. This omission is crucial: aggregating effect sizes from studies of vastly different rigor without weighting for quality undermines the validity of the overall findings.
Moreover, the authors do not explore heterogeneity – despite it being high. In a robust meta-analysis, differences in context, methodology, or sample characteristics would be analyzed to understand where effects differ and why. Wang & Fan ignore this, presenting average effect sizes as if they describe a single, consistent phenomenon.
Misinterpretation Risks: What Does g = 0.867 Actually Mean?
The headline claim of the study is that ChatGPT improves student learning performance with a large effect size (g = 0.867). This is statistically large according to standard benchmarks:
g Value | Interpretation |
---|---|
0.2 | Small effect |
0.5 | Moderate effect |
0.8+ | Large effect |
But here’s the catch: an impressive effect size is meaningless if based on flawed or incomparable inputs.
For example, some included studies appear to:
- Lack control groups
- Use teacher-made or unvalidated assessments
- Have treatment groups with fewer than 35 students
- Come from low-credibility journals with limited peer review
A value like g = 0.867, calculated across such varied studies, might look precise but tells us little about real-world, generalizable effects.
Misleading Generalizations by Context
1. Best Use in STEM and Skill-Based Courses?
The study reports:
- STEM subjects: g = 0.737
- Skills courses: g = 0.874
These figures are presented as if AI reliably boosts structured learning. However, the lack of consistency across studies (e.g., task type, assessment format, duration) means these results may reflect cherry-picked effects or publication bias more than genuine insight.
2. High Impact in Problem-Based Learning?
The reported g = 1.113 in problem-based learning is the largest figure in the study. But no explanation is given about how many studies fall into this category, how PBL was defined, or whether comparison groups followed similar pedagogical models. Again, the result is eye-catching but analytically weak.
3. Ideal Duration: 4–8 Weeks?
The study claims that interventions lasting 4–8 weeks yielded the best results (g = 0.999). But no statistical justification is offered for why this window matters. It’s likely that longer exposure simply allowed students more time to adapt – yet shorter durations could reflect pilot programs or single-session trials, which are not directly comparable.
Support for Higher-Order Thinking?
Wang & Fan report a moderate effect on critical thinking (g = 0.457) and suggest that using ChatGPT as a tutor (g = 0.945) or with scaffolds like Bloom’s taxonomy enhances outcomes. While plausible, these claims again rest on uncategorized and unverified implementations, lacking evidence that higher-order skills were actually measured through validated instruments.
Why These Effect Sizes Lack Reliability
To illustrate, suppose a ChatGPT group scores 85 on a test, and a control group scores 75. If the pooled standard deviation is 13.5, then:
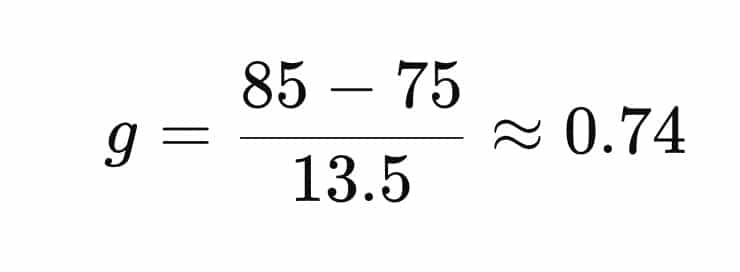
This would suggest a “large” effect. But what if:
- The test was made by the teacher?
- The intervention lasted one hour?
- The control group used outdated materials?
In the absence of quality filtering, even accurate math yields inaccurate meaning.
Summary Table: Results Without Context Don’t Equal Truth
Context / Variable | Effect Size (g) | Critical View |
---|---|---|
Overall Learning Performance | 0.867 | High, but based on weak study pool |
Higher-Order Thinking | 0.457 | Moderate, but conceptually vague |
Student Perception | 0.456 | Moderate, based on self-report |
STEM Subjects | 0.737 | Validity unclear |
Skill Courses | 0.874 | No clarity on course type |
Language Learning | 0.334 | Poor outcome, yet not explored |
Problem-Based Learning | 1.113 | No definition or controls given |
4–8 Week Use Duration | 0.999 | May reflect adaptation period |
Complete Lack of Methodological Control,
The Wang & Fan (2025) study illustrates the dangers of quantifying educational interventions without rigor. While the use of Hedges’ g gives the illusion of precision, the lack of methodological control, inconsistent definitions, and absence of quality thresholds mean that these numbers offer little actionable insight.
Educators, policymakers, and researchers should not take the reported results at face value. Instead, they must demand higher standards in AI-in-education research – where transparency, theory, and replicability matter as much as effect size.